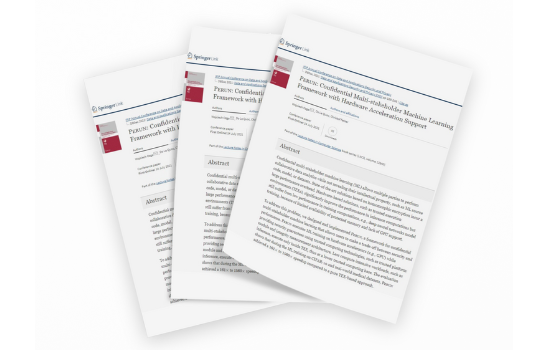
Abstract
Confidential multi-stakeholder machine learning (ML) allows multiple parties to perform collaborative data analytics while not revealing their intellectual property, such as ML source code, model, or datasets. State-of-the-art solutions based on homomorphic encryption incur a large performance overhead. Hardware-based solutions, such as trusted execution environments (TEEs), significantly improve the performance in inference computations but still suffer from low performance in training computations, e.g., deep neural networks model training, because of limited availability of protected memory and lack of GPU support.
To address this problem, we designed and implemented PERUN, a framework for confidential multi-stakeholder machine learning that allows users to make a trade-off between security and performance. PERUN executes ML training on hardware accelerators (e.g., GPU) while providing security guarantees using trusted computing technologies, such as trusted platform module and integrity measurement architecture. Less compute-intensive workloads, such as inference, execute only inside TEE, thus at a lower trusted computing base. The evaluation shows that during the ML training on CIFAR-10 and real-world medical datasets, PERUN achieved a 161× to 1560× speedup compared to a pure TEE-based approach.
Authors
- Wojciech Ozga, IBM, Switzerland.
- Do Le Quoc, Technische Universität Dresden, Germany.
- Christof Fetzer, Technische Universität Dresden, Germany.
Keywords
Multi-stakeholder computation, Machine learning, Confidential computing, Trusted computing, Trust management